Predicting Fisheries Collapse
By: Chris Schenker, SRC Intern
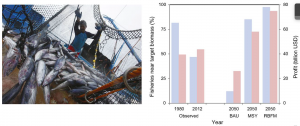
Figure 1: Some fisheries, such as skipjack tuna (left) remain profitable. Shown to the right, blue bars indicate fisheries that remain close to target biomass, while red bars indicate fisheries that generate profit. Predictions for 2050 are shown based on business as usual (BAU), maximum sustainable yield through catch limits (MSY), and maximum profit through rights-based fisheries management (RBFM). (Worm et al., 2009)
Hundreds of millions of people around the world depend on wild harvests from the ocean as both a livelihood and source of protein (Srinivasan et al., 2010). Ensuring the long term sustainability of fisheries is therefore a matter of utmost importance for global resource security. However, according to a 2016 paper in PNAS, global fish stocks are on average poor and declining (Costello et al., 2016). Of the 4,714 fish stocks analyzed by Costello et al. in 2012, 68% were below the biomass target that supports maximum sustainable yield (referred to as BMSY). Considering a level of 63% of assessed stocks measuring below BMSY in 2006 (Worm et al., 2009), there has been a clear decrease in fisheries health worldwide. Furthermore, the finding that only 35% of stocks in decline are being fished at a rate that puts them back on target to achieve BMSY indicates that this trend is set to worsen (Costello et al., 2016).
In order to avert a collapse in the world’s fisheries, it is therefore important to understand which species are at greater risk than others. Terrestrial surveys suggest that large bodied, high trophic level species are the most susceptible to population decline due to human pressure. While this was long assumed to also be the case for marine species, recent evidence has suggested otherwise. Pinsky et al. analyzed two independent fisheries databases and global landings from 1950 to 2006 as reported by the UN Food and Agriculture Organization (FAO) in order to test this hypothesis (Pinsky et al., 2011).
Neither the assessment data nor the landings data supported the idea that fisheries collapse across only a small range of life history traits. In the analysis of the assessment data, 12% of stocks of high trophic level species experienced collapse, but 25% had collapsed among low trophic level species. When comparing by size, 16% of large species collapsed versus 29% of small species. While these figures suggest a higher likelihood of collapse in smaller, low trophic level species, linear regression models did not support any significant relationship between stock collapse and trophic level (P = 0.15), weight (P = 0.26), longevity (P = 0.10), age of maturity (P = 0.92), fecundity (P = 0.77), or investment in offspring (P = 0.99). The landings data supported the conclusion that small, low trophic level species are just as vulnerable as large, top predators, and individual life history traits alone did not explain significant variation in stock collapse (Pinksy et al., 2011).
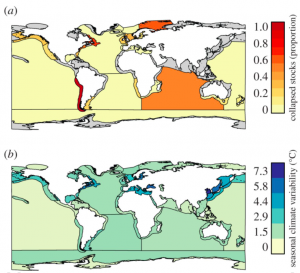
Figure 2: Global variation by large marine ecosystem in (a) proportion of stocks that have ever collapsed, and (b) climate variability from season to season. (Pinsky et al., 2015)
In a 2015 paper, Pinsky et al. created boosted regression trees for 154 populations of fish worldwide in order to analyze the effects of harvesting, life history traits and climate variability on the risk of collapse. As expected, overfishing was the most important predictor of collapse. Chronic overfishing correlated to depletion, while acute overfishing correlated to collapse (Pinsky & Byler 2015). However, certain life history traits and climate variability were found to predispose populations for collapse.
Fast-growing species in variable climates appeared particularly sensitive to overfishing. Species with short generation times can rebound faster than longer generation species, but they can only tolerate smaller lags in harvest rate reductions. When coupled with productivity lows caused by climate variability, fast growing species become difficult to manage and require proactive oversight. There are limitations to the datasets used to arrive at this conclusion, such as the low number of tropical climates assessed, but it is clear that more variability exists than can be explained by simple extinction models (Lande, 1993). More complex models incorporating multiple, interacting drivers of stock declines appear useful for better predicting future collapses.
Stock assessments are an important component of most management systems, but their prohibitive cost often means they can only be completed infrequently. It is therefore imperative to maximize the value of an assessment by deriving as much information about as many species as possible. In PNAS, Burgess et al. (Burgess et al. 2013) suggest a new system for predicting future population declines. The theory assumes that the species in any multispecies fishery are connected to each other by the shared threat of effort. This means that the fate of all species can be predicted if the fate of one species can be predicted.
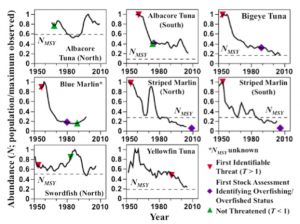
Figure 3: Comparison of assessment histories and points of earliest identifiable threat based on T-score. (Burgess et al., 2013)
The logic behind this assumption is that in any fishery, most of the harvesting will be directed at key species, and this will drive future mortality from fishing for all species. Target species are usually monitored more consistently and have more extensive datasets of past and present stocks (Burgess et al., 2013). By using differences in life history traits between key and other species and projections of future mortality from fishing, the authors created a T-score or “eventual threat index” for any given species across all of the fisheries in which it is caught. A T-score < 1 means a species is not at risk, while greater than one means a weak stock is on track to become overfished. Once T increases to > 2, the species is at risk to become extinct due to incidental fishing pressure.
This new approach promises to boost the effectiveness of fisheries management worldwide. Eight populations of Pacific tuna and billfish were analyzed with this technique, four of which are severely depleted. Had this method been available then, the authors claim that the decline of these populations could have been predicted in the 1950’s (Burgess et al., 2013). Such early detection could have positive economic impact and save decades in recovery time. However, alternative solutions are still needed in less developed countries lacking sufficient assessment and regulation. If depleted fish populations in these countries are ever going to recover, new sources of income and nutrition must be found to supplement the role that fishing has traditionally filled. Saving global fisheries from collapse is possible, but it will require innovative new science, strict regulation, and engagement with less developed communities that rely on the sea for livelihood.
Works Cited:
Burgess MG, Polasky S, Tilman D (2013) Predicting overfishing and extinction threats in multispecies fisheries. Proc Natl Acad Sci USA110:15943–15948.
Costello C, et al. (2016) Global fishery prospects under contrasting management regimes. Proc Natl Acad Sci USA 113:5125–5129.
Lande R. 1993 Risks of population extinction from demographic and environmental stochasticity and random catastrophes. Am. Nat. 142, 911–927
Pinsky ML, Jensen O. P. , Ricard D., Palumbi S. R. 2011 Unexpected patterns of fisheries collapse in the world’s oceans. Proc. Natl. Acad. Sci. U.S.A. 108, 8317–8322. doi: 10.1073/pnas.1015313108; pmid: 21536889
Pinsky ML, Byler D. 2015. Fishing, fast growth and climate variability increase the risk of collapse. Proc. R. Soc. B 282:20151053
Srinivasan, U.T., Cheung, W.W.L., Watson, R. et al. J Bioecon (2010) 12: 183. doi: 10.1007/s10818-010-9090-9
Worm B, et al. (2009) Rebuilding global fisheries. Science 325(5940):578–585.